Generative AI describes deep learning models that can learn from raw data and generate new outputs based on learned patterns. Previously confined to analyzing numerical data, generative models made significant strides with the advent of deep learning. Variational autoencoders (VAEs), introduced in 2013, were pioneers in this domain, enabling the generation of realistic images and speech. VAEs laid the foundation for the rapid development of technologies like generative adversarial networks (GANs) and diffusion models, which produce increasingly realistic but synthetic content.
Central to the progress of generative AI is the encoder-decoder architecture, which also forms the backbone of large language models. Encoders compress data into dense representations, grouping similar data points, while decoders use these representations to generate new content.
Architecture
In 2017, Google introduced transformers, combining the encoder-decoder architecture with an attention mechanism, revolutionizing language model training. Encoders convert raw text into embeddings, and decoders predict each word in a sentence using embeddings and prior model outputs. This fill-in-the-blank approach allows transformers to grasp language nuances and grammar without manual annotations.
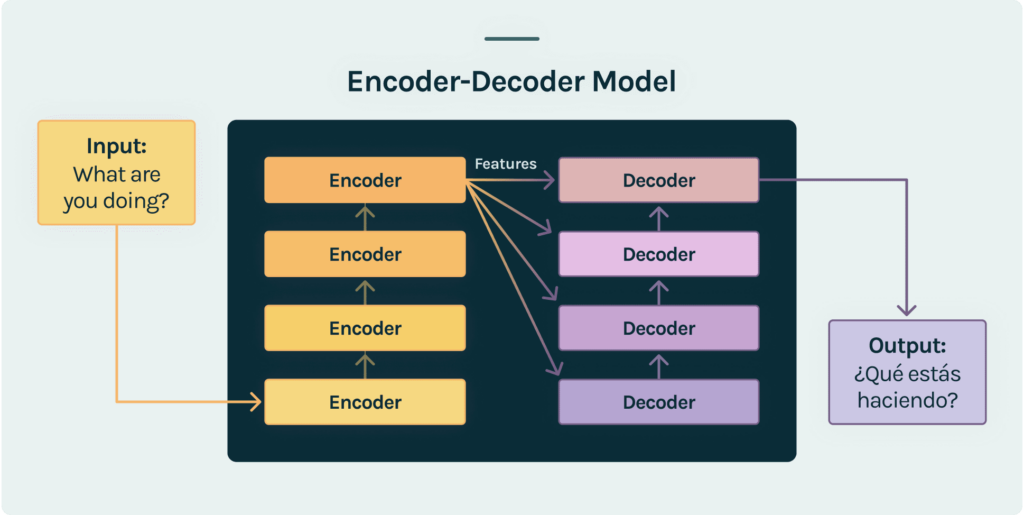
Transformers’ versatility allows them to be pre-trained on extensive raw text and later fine-tuned for specific tasks with minimal labeled data. Three primary categories of language transformers emerged: encoder-only, decoder-only, and encoder-decoder models. Encoder-only models like BERT excel at classification and extraction tasks, while decoder-only models like GPT specialize in generating text. Encoder-decoder models like a neural machine translation system combine features of both, offering a balance between generative capability and computational efficiency.
Supervised learning, once overshadowed by unsupervised learning, has seen a resurgence in shaping interactions with generative models. Instruction-tuning, zero-shot learning, and few-shot learning enable users to instruct models for specific tasks, even without labeled data. Techniques like prompt-tuning and adaptors empower users to customize models using proprietary data without extensive fine-tuning.
Human supervision has further propelled generative AI, aligning model behavior with human preferences. Reinforcement learning from human feedback (RLHF) fine-tunes models based on human-rated responses, leading to AI systems producing high-quality conversational text.
As generative AI advances, the question of model size and specialization arises. While larger models initially dominated, smaller, domain-specialized models have proven effective for specific tasks. The concept of model distillation also challenges the necessity of large models, as researchers successfully imbue smaller models with similar capabilities.
Applications
Here are a few key areas where this technology is already making significant strides:
- Content generation: Generative AI has revolutionized content creation. From writing articles to generating marketing campaigns, AI-powered tools can assist writers by producing drafts with various styles that can then be refined and customized.
- Art and design: The application of AI in the domain of art and design has produced impressive creations. Artists are using Generative AI to create visual pieces, and designers are leveraging its capabilities to generate novel design concepts. This technology serves as a wellspring of inspiration, pushing the boundaries of human creativity.
- Healthcare and drug Discovery: In the healthcare sector, Generative AI is assisting researchers in drug discovery. By analyzing vast datasets of molecular structures and pharmacological data, AI can propose potential drug candidates with a higher likelihood of success. This accelerates the drug development process.
- Personalized marketing: Generative AI is reshaping marketing strategies by enabling the creation of hyper-personalized content. By analyzing customer behaviors and preferences, AI can generate tailored product recommendations and marketing messages, enhancing customer engagement and conversion rates.
- Video game development: In the realm of gaming, Generative AI is being used to design virtual environments, characters, and even entire game levels. This not only reduces the time and resources required for development but also introduces unexpected and innovative elements to gameplay.
To learn more about how to build, deploy, and operate high-quality generative AI applications read the other articles in this section.