Trustworthy AI in Insurance
Explain, analyse, and monitor ML models. Improve quality. Build trust. Scale up
Scaling AI in Insurance
Artificial Intelligence and Machine Learning (AI/ML) has the potential to transform insurance, touching every core process: marketing, underwriting, pricing, claims, fraud management, customer engagement, and investment management.
However, real-world adoption of AI/ML remains sub-scale in most insurers. For many, AI/ML models are not considered transparent and reliable enough to meet business, customer and regulatory expectations in high-stakes use cases.
Insurers face a difficult choice – ride the AI/ML tiger and risk reputational and regulatory blowback from poorly managed risks, or continue with lukewarm adoption and cede ground to ‘braver’ tech-first challengers. They must take the challenge head-on, and put in tools and processes to make AI trustworthy. TruEra’s AI Quality solutions help explain, analyze and monitor AI/ML models – to ensure quality, build trust and enable impact at scale.
Barriers to AI Adoption

Explainability
Is the regulator able to understand how customers’ data is used for underwriting & pricing decisions?

Data Bias
Will the pricing model trained using data from one geography or segment work in another?

Fairness
Are claims made by customers of a particular race, gender or age group more likely to be discriminated against?

Stability
How did the insurance underwriting model react to a major environmental change? Is the model still valid?
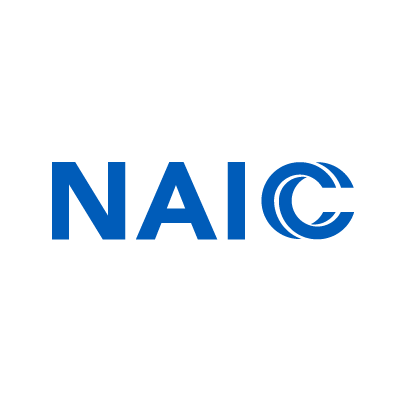
Insurers and relevant third parties “should apply a systematic risk management approach to each phase of the AI system life cycle on a continuous basis to address risks related to AI systems, including privacy, digital security and unfair discrimination as defined by applicable laws and regulations.”
US National Association of Insurance Commissioners (NAIC) Principles on AI, Aug 2020
TruEra helps insurers and asset managers capture real business value from AI/ ML

Faster deployment
Improve model quality earlier in the lifecycle. Accelerate time to approval.

Greater buy-in
Easily explain models to stakeholders. Build understanding and trust.

Robust governance
Automate compliance with regulatory and internal standards on AI/ ML use.
Use cases in insurance
Underwriting
- Understand what drives underwriting models’ decisions as to which classes to write, along with which terms & conditions to apply
- Understand what drives underwriting models’ decisions as to which classes to write, along with which terms & conditions to apply
Pricing
- Confirm pricing models are not using – either directly or as a proxy – data points that are not regulatory compliant
- Obtain regulatory approval for pricing models by clearly explaining how premiums are being calculated by the model
Claims management
- Monitor the predictive power and stability of the claims triage model on an ongoing basis
- Help investigators understand why particular claims have been flagged as fraudulent
Targeted Marketing
- Assess whether mvarketing approach is promoting products unsuitable to customers’ circumstances and/ or needs
- Determine whether certain segments, however defined, are being actively, possibly inappropriately, targeted or avoided
Investment process
- Understand drivers of investment models, thereby identifying potential biases
- Monitor the reliability of investment recommendations
Operations Automation
- Provide early warnings when data drift is likely to impact the accuracy of models used to automate back-office processes
- Diagnose quickly root causes for performance degradation in operational efficiency models